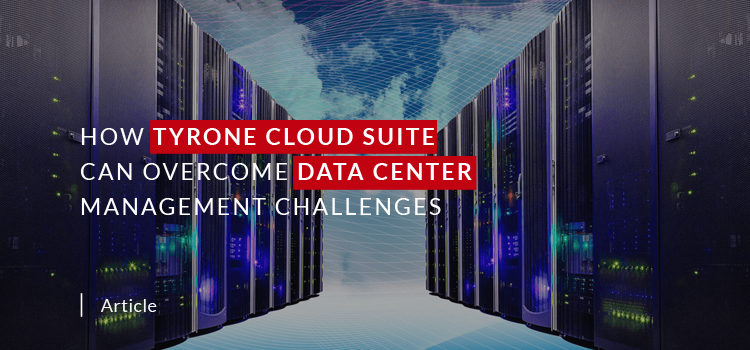
The emergence of digital transformation has made organizations realize the importance of data in making strategies, analyzing market trends, creating better experiences for customers, and finding out ways to stay ahead from their competitors. Data is becoming the key to competitive advantage.
However, the data that is being generated after technological advancements is way more different and evolved from the earlier one in terms of transactions, structure, availability, methods of collection, and value derived from the ability to aggregate and analyze it. The data is extensive and can easily dominate any aspect of business decision making. To make it usable in a cohesive environment, it can be divided into two categories – big data and fast data.
Big data is large collected data sets used for batch analytics, whereas, fast data is collected from many sources and is used to drive immediate decision making. Despite having advanced ways of storing and using this data, there are some challenges that data centers face and needs to be addressed for the effective utilization of data. Let’s check them out.
The data centers have a lot of applications, connecting cables, network connectivity, cooling systems, power distribution, storage units, and much more things running at the same time. This heavy load could lead to unexpected failures. Therefore, constant monitoring and reporting different metrics becomes a must-do for data center operators and managers.
Data center managers tend to overprovision to avoid downtimes. As a result, resources, space, power and energy is wasted. The increase in data has always questioned the capacity of a data center making it a challenge for data center managers. However, this was only till data center infrastructure management solution came into being.
One of the major concerns for data center managers and operators is to measure the performance and ensure uptime. This includes maintaining power and cooling accuracy ensuring energy efficiency of the overall structure too. In such cases, any manual management is simply cost-prohibitive at the scale that they operate at.
he activities of data center infrastructure involves tracking, analyzing and reporting performances which if done by non-automated or manual systems need facilities and IT staff to spend an extraordinary amount of time logging activities into spreadsheets. This can become a hindrance in the time that can be spent making strategic decisions for improving data center services.
The data center is estimated to account for 1.4% of the global electricity consumption. The industry is often accused of using massive amount of energy and rising temperature problem. There are times when energy is found to be wasted than used at a data center site. This is due to lack of proper energy monitoring tools and environmental sensors.
Cognitive computing
Machine learning in the cloud does exactly this. The large amounts of data stored in the cloud provide a source of information for the machine learning process. With millions of people using the cloud for computing, storage and networking, the already existing data, the millions of processes that happen every day, all provide a source of information for the machine to learn from. The whole process will provide applications in the cloud with sensory capabilities. The applications will be able to perform cognitive functions and make decisions.
Some examples of cognitive computing in the current market have made remarkable progress in the field of artificial intelligence. IBM Watson, AWS IA, and Microsoft Cognitive APIs have been notable cases in the industry.
Some examples of cognitive computing in the current market have made remarkable progress in the field of artificial intelligence. IBM Watson, AWS IA, and Microsoft Cognitive APIs have been notable cases in the industry.
Cognitive computing systems in existence today are more at an experimental stage and are given tasks of minimal importance. Over time, we can expect these systems to take over healthcare and hospitality, business and personal lives even.
Cognitive computing
Machine learning in the cloud does exactly this. The large amounts of data stored in the cloud provide a source of information for the machine learning process. With millions of people using the cloud for computing, storage and networking, the already existing data, the millions of processes that happen every day, all provide a source of information for the machine to learn from. The whole process will provide applications in the cloud with sensory capabilities. The applications will be able to perform cognitive functions and make decisions.
Some examples of cognitive computing in the current market have made remarkable progress in the field of artificial intelligence. IBM Watson, AWS IA, and Microsoft Cognitive APIs have been notable cases in the industry.
Cognitive computing systems in existence today are more at an experimental stage and are given tasks of minimal importance. Over time, we can expect these systems to take over healthcare and hospitality, business and personal lives even.
Personal assistance and chatbots
Personal assistants have made life easier for individuals. Products like Apple Siri, Google Allo, or Microsoft Cortana are pre-coded voice recognition systems that give a feel of human touch to machines. But these personal digital assistants have limited capabilities. With the mass data on the cloud, the learning capabilities of machine learning, and its cognitive computing feature as mentioned above, personal assistance can almost replace any form of human interaction. Fantasies of owning computer systems like those in science fiction or super-hero movies can become a reality.
mplementing machine learning will increase the cognitive capabilities of these chatbots, giving them a human touch. These chatbots can learn from past conversations and provide better assistance. Not just that, instead of a plain, question-answer session between the customer and the chatbot, a real conversation can take place with the chatbot. The chatbot can initiate queries about previous problems or additional suggestions for the problem at hand. The main aim is to make these chatbots as human and personal as possible to make customers feel important.